Estimating Demand for Taxis at LaGuardia Airport
Capstone Project for Columbia University M.S. in Data Science
There is often a mismatch between the demand and supply of taxis at New York City's LaGuardia Airport (LGA). This can lead to long wait times for passengers in the taxi queues and for drivers in the taxi hold lots. Better predictions of taxi demand could improve demand-supply balance and, as a result, passenger and driver satisfaction.
For our thesis project, three fellow Columbia Data Science M.S. students and I had the opportunity to work with New York City's Taxi and Limousine Commission (TLC). We trained several machine learning models to predict the hourly number of taxi pickups at LGA. We implemented and evaluated an ensemble of tree-based models, achieving a Mean-Absolute Error (MAE) of 56.9 and coefficient of determination (R2) of 0.908. We also implemented a Long Short-Term Memory (LSTM) Recurrent Neural Network, which achieved an MAE of 48.1 and R2 of 0.921.
We trained our models using publicly available data from the TLC, Bureau of Transportation Statistics (BTS), and many other sources outlined in our paper.
Below you can see our neural network's performance over six months' of testing data from January 2017 through June 2017.
We had the pleasure of presenting our work to New York City's Taxi and Limousine Commission.
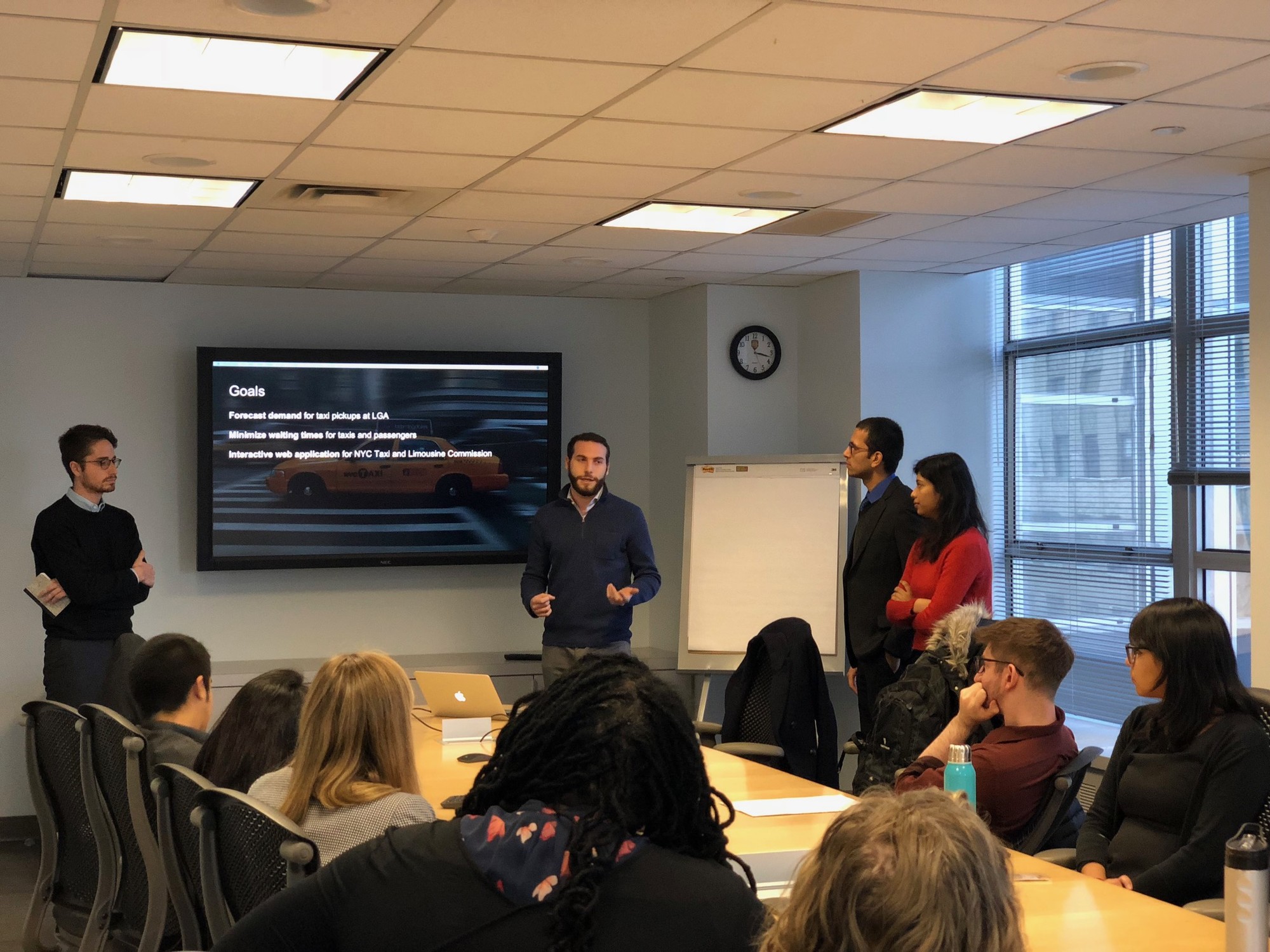
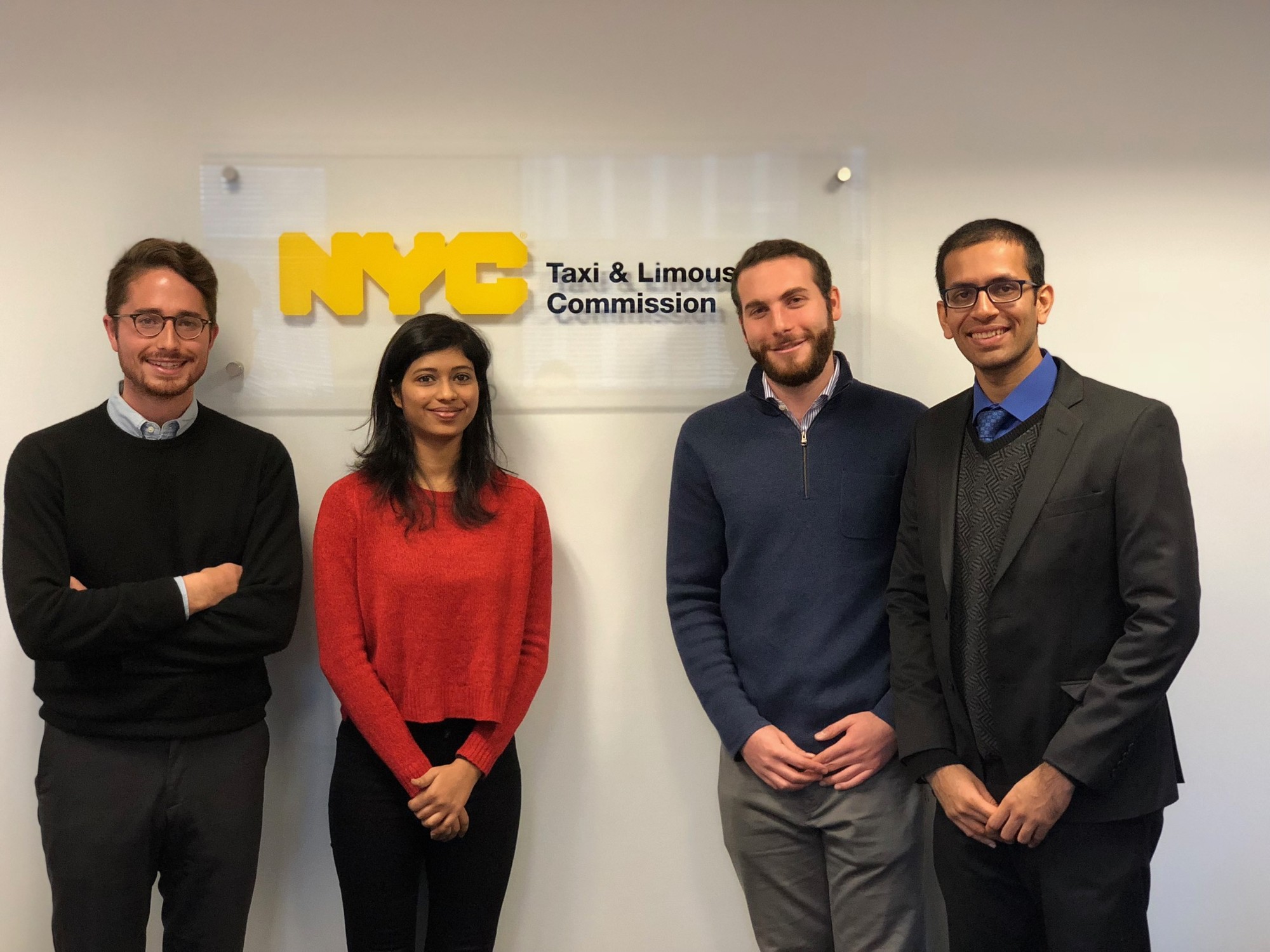
Thank you to Columbia's Data Science Institute and NYC's Taxi and Limousine Commission for the opportunity to work on this project.